1
2
3
4
5
6
7
8
9
10
11
12
13
14
15
16
17
18
19
20
21
22
23
24
25
26
27
28
29
30
31
32
33
34
35
36
37
38
39
40
41
42
43
44
45
46
47
48
49
50
51
52
53
54
55
56
57
58
59
60
61
62
63
64
65
66
67
68
69
70
71
72
73
74
75
76
77
78
79
80
81
82
83
84
85
86
87
88
89
90
91
92
93
94
95
96
97
98
99
100
101
102
103
104
105
106
107
108
109
110
111
112
113
114
115
116
117
118
119
120
121
122
123
124
125
126
127
128
129
130
131
132
133
134
135
136
137
138
139
140
141
142
143
144
145
146
147
148
149
150
151
152
153
154
155
156
157
158
159
160
161
162
163
164
165
166
167
168
169
170
171
172
173
174
175
176
177
178
179
180
181
182
183
184
185
186
187
188
189
190
191
192
193
194
195
196
197
198
199
200
201
202
203
204
205
206
207
208
209
210
211
212
213
214
215
216
217
218
219
220
221
222
223
224
225
226
227
228
229
230
231
232
233
234
235
236
237
238
239
240
241
242
243
244
245
246
247
248
249
250
251
252
253
254
255
256
257
258
259
260
261
262
263
264
265
266
267
268
269
270
271
272
273
274
275
276
277
278
279
280
281
282
283
284
285
286
287
288
289
290
291
292
293
294
295
296
297
298
299
300
301
302
303
304
305
306
307
308
309
310
311
312
313
314
315
316
317
318
319
320
321
322
323
324
325
326
327
328
329
330
331
332
333
334
335
336
337
338
339
340
341
342
343
344
345
346
347
348
349
350
351
352
353
354
355
356
357
358
359
360
361
362
363
364
365
366
367
368
369
370
371
372
373
374
375
376
377
378
379
380
381
382
383
384
385
386
387
388
389
390
391
392
393
394
395
396
397
398
399
400
401
402
403
404
405
406
407
408
409
410
411
412
413
414
415
416
417
418
419
420
421
422
423
424
425
426
427
428
429
430
431
432
433
434
435
436
437
438
439
440
441
442
443
444
445
446
447
448
449
450
451
452
453
454
455
456
457
458
459
460
461
462
463
464
465
466
467
468
469
470
471
472
473
474
475
476
477
478
479
480
481
482
483
484
485
486
487
488
489
490
491
492
493
494
495
496
497
498
499
500
501
502
503
504
505
506
507
508
509
510
511
512
513
514
515
516
517
518
519
520
521
522
523
524
525
526
527
528
529
530
531
532
533
534
535
536
537
538
539
540
541
542
543
544
545
546
547
548
549
550
551
552
553
554
555
556
557
558
559
560
561
562
563
564
565
566
567
568
569
570
571
572
573
574
575
576
577
578
579
580
581
582
583
584
585
586
587
588
589
590
591
592
593
594
595
596
597
598
599
600
601
602
603
604
|
# Introduction
This guide gets you started programming in the low-level TensorFlow APIs
(TensorFlow Core), showing you how to:
* Manage your own TensorFlow program (a `tf.Graph`) and TensorFlow
runtime (a `tf.Session`), instead of relying on Estimators to manage them.
* Run TensorFlow operations, using a `tf.Session`.
* Use high level components ([datasets](#datasets), [layers](#layers), and
[feature_columns](#feature_columns)) in this low level environment.
* Build your own training loop, instead of using the one
@{$premade_estimators$provided by Estimators}.
We recommend using the higher level APIs to build models when possible.
Knowing TensorFlow Core is valuable for the following reasons:
* Experimentation and debugging are both more straight forward
when you can use low level TensorFlow operations directly.
* It gives you a mental model of how things work internally when
using the higher level APIs.
## Setup
Before using this guide, @{$install$install TensorFlow}.
To get the most out of this guide, you should know the following:
* How to program in Python.
* At least a little bit about arrays.
* Ideally, something about machine learning.
Feel free to launch `python` and follow along with this walkthrough.
Run the following lines to set up your Python environment:
```python
from __future__ import absolute_import
from __future__ import division
from __future__ import print_function
import numpy as np
import tensorflow as tf
```
## Tensor Values
The central unit of data in TensorFlow is the **tensor**. A tensor consists of a
set of primitive values shaped into an array of any number of dimensions. A
tensor's **rank** is its number of dimensions, while its **shape** is a tuple
of integers specifying the array's length along each dimension. Here are some
examples of tensor values:
```python
3. # a rank 0 tensor; a scalar with shape [],
[1., 2., 3.] # a rank 1 tensor; a vector with shape [3]
[[1., 2., 3.], [4., 5., 6.]] # a rank 2 tensor; a matrix with shape [2, 3]
[[[1., 2., 3.]], [[7., 8., 9.]]] # a rank 3 tensor with shape [2, 1, 3]
```
TensorFlow uses numpy arrays to represent tensor **values**.
## TensorFlow Core Walkthrough
You might think of TensorFlow Core programs as consisting of two discrete
sections:
1. Building the computational graph (a `tf.Graph`).
2. Running the computational graph (using a `tf.Session`).
### Graph
A **computational graph** is a series of TensorFlow operations arranged into a
graph. The graph is composed of two types of objects.
* `tf.Operation` (or "ops"): The nodes of the graph.
Operations describe calculations that consume and produce tensors.
* `tf.Tensor`: The edges in the graph. These represent the values
that will flow through the graph. Most TensorFlow functions return
`tf.Tensors`.
Important: `tf.Tensors` do not have values, they are just handles to elements
in the computation graph.
Let's build a simple computational graph. The most basic operation is a
constant. The Python function that builds the operation takes a tensor value as
input. The resulting operation takes no inputs. When run, it outputs the
value that was passed to the constructor. We can create two floating point
constants `a` and `b` as follows:
```python
a = tf.constant(3.0, dtype=tf.float32)
b = tf.constant(4.0) # also tf.float32 implicitly
total = a + b
print(a)
print(b)
print(total)
```
The print statements produce:
```
Tensor("Const:0", shape=(), dtype=float32)
Tensor("Const_1:0", shape=(), dtype=float32)
Tensor("add:0", shape=(), dtype=float32)
```
Notice that printing the tensors does not output the values `3.0`, `4.0`, and
`7.0` as you might expect. The above statements only build the computation
graph. These `tf.Tensor` objects just represent the results of the operations
that will be run.
Each operation in a graph is given a unique name. This name is independent of
the names the objects are assigned to in Python. Tensors are named after the
operation that produces them followed by an output index, as in
`"add:0"` above.
### TensorBoard
TensorFlow provides a utility called TensorBoard. One of TensorBoard's many
capabilities is visualizing a computation graph. You can easily do this with
a few simple commands.
First you save the computation graph to a TensorBoard summary file as
follows:
```
writer = tf.summary.FileWriter('.')
writer.add_graph(tf.get_default_graph())
```
This will produce an `event` file in the current directory with a name in the
following format:
```
events.out.tfevents.{timestamp}.{hostname}
```
Now, in a new terminal, launch TensorBoard with the following shell command:
```bsh
tensorboard --logdir .
```
Then open TensorBoard's [graphs page](http://localhost:6006/#graphs) in your
browser, and you should see a graph similar to the following:
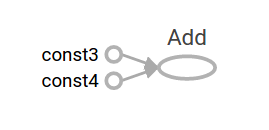
For more about TensorBoard's graph visualization tools see @{$graph_viz}.
### Session
To evaluate tensors, instantiate a `tf.Session` object, informally known as a
**session**. A session encapsulates the state of the TensorFlow runtime, and
runs TensorFlow operations. If a `tf.Graph` is like a `.py` file, a `tf.Session`
is like the `python` executable.
The following code creates a `tf.Session` object and then invokes its `run`
method to evaluate the `total` tensor we created above:
```python
sess = tf.Session()
print(sess.run(total))
```
When you request the output of a node with `Session.run` TensorFlow backtracks
through the graph and runs all the nodes that provide input to the requested
output node. So this prints the expected value of 7.0:
```
7.0
```
You can pass multiple tensors to `tf.Session.run`. The `run` method
transparently handles any combination of tuples or dictionaries, as in the
following example:
```python
print(sess.run({'ab':(a, b), 'total':total}))
```
which returns the results in a structure of the same layout:
``` None
{'total': 7.0, 'ab': (3.0, 4.0)}
```
During a call to `tf.Session.run` any `tf.Tensor` only has a single value.
For example, the following code calls `tf.random_uniform` to produce a
`tf.Tensor` that generates a random 3-element vector (with values in `[0,1)`):
```python
vec = tf.random_uniform(shape=(3,))
out1 = vec + 1
out2 = vec + 2
print(sess.run(vec))
print(sess.run(vec))
print(sess.run((out1, out2)))
```
The result shows a different random value on each call to `run`, but
a consistent value during a single `run` (`out1` and `out2` receive the same
random input):
```
[ 0.52917576 0.64076328 0.68353939]
[ 0.66192627 0.89126778 0.06254101]
(
array([ 1.88408756, 1.87149239, 1.84057522], dtype=float32),
array([ 2.88408756, 2.87149239, 2.84057522], dtype=float32)
)
```
Some TensorFlow functions return `tf.Operations` instead of `tf.Tensors`.
The result of calling `run` on an Operation is `None`. You run an operation
to cause a side-effect, not to retrieve a value. Examples of this include the
[initialization](#Initializing Layers), and [training](#Training) ops
demonstrated later.
### Feeding
As it stands, this graph is not especially interesting because it always
produces a constant result. A graph can be parameterized to accept external
inputs, known as **placeholders**. A **placeholder** is a promise to provide a
value later, like a function argument.
```python
x = tf.placeholder(tf.float32)
y = tf.placeholder(tf.float32)
z = x + y
```
The preceding three lines are a bit like a function in which we
define two input parameters (`x` and `y`) and then an operation on them. We can
evaluate this graph with multiple inputs by using the `feed_dict` argument of
the `tf.Session.run` method to feed concrete values to the placeholders:
```python
print(sess.run(z, feed_dict={x: 3, y: 4.5}))
print(sess.run(z, feed_dict={x: [1, 3], y: [2, 4]}))
```
This results in the following output:
```
7.5
[ 3. 7.]
```
Also note that the `feed_dict` argument can be used to overwrite any tensor in
the graph. The only difference between placeholders and other `tf.Tensors` is
that placeholders throw an error if no value is fed to them.
## Datasets
Placeholders work for simple experiments, but `tf.data` are the
preferred method of streaming data into a model.
To get a runnable `tf.Tensor` from a Dataset you must first convert it to a
`tf.data.Iterator`, and then call the Iterator's
`tf.data.Iterator.get_next` method.
The simplest way to create an Iterator is with the
`tf.data.Dataset.make_one_shot_iterator` method.
For example, in the following code the `next_item` tensor will return a row from
the `my_data` array on each `run` call:
``` python
my_data = [
[0, 1,],
[2, 3,],
[4, 5,],
[6, 7,],
]
slices = tf.data.Dataset.from_tensor_slices(my_data)
next_item = slices.make_one_shot_iterator().get_next()
```
Reaching the end of the data stream causes `Dataset` to throw an
`tf.errors.OutOfRangeError`. For example, the following code
reads the `next_item` until there is no more data to read:
``` python
while True:
try:
print(sess.run(next_item))
except tf.errors.OutOfRangeError:
break
```
If the `Dataset` depends on stateful operations you may need to
initialize the iterator before using it, as shown below:
``` python
r = tf.random_normal([10,3])
dataset = tf.data.Dataset.from_tensor_slices(r)
iterator = dataset.make_initializable_iterator()
next_row = iterator.get_next()
sess.run(iterator.initializer)
while True:
try:
print(sess.run(next_row))
except tf.errors.OutOfRangeError:
break
```
For more details on Datasets and Iterators see: @{$guide/datasets}.
## Layers
A trainable model must modify the values in the graph to get new outputs with
the same input. `tf.layers` are the preferred way to add trainable
parameters to a graph.
Layers package together both the variables and the operations that act
on them. For example a
[densely-connected layer](https://developers.google.com/machine-learning/glossary/#fully_connected_layer)
performs a weighted sum across all inputs
for each output and applies an optional
[activation function](https://developers.google.com/machine-learning/glossary/#activation_function).
The connection weights and biases are managed by the layer object.
### Creating Layers
The following code creates a `tf.layers.Dense` layer that takes a
batch of input vectors, and produces a single output value for each. To apply a
layer to an input, call the layer as if it were a function. For example:
```python
x = tf.placeholder(tf.float32, shape=[None, 3])
linear_model = tf.layers.Dense(units=1)
y = linear_model(x)
```
The layer inspects its input to determine sizes for its internal variables. So
here we must set the shape of the `x` placeholder so that the layer can
build a weight matrix of the correct size.
Now that we have defined the calculation of the output, `y`, there is one more
detail we need to take care of before we run the calculation.
### Initializing Layers
The layer contains variables that must be **initialized** before they can be
used. While it is possible to initialize variables individually, you can easily
initialize all the variables in a TensorFlow graph as follows:
```python
init = tf.global_variables_initializer()
sess.run(init)
```
Important: Calling `tf.global_variables_initializer` only
creates and returns a handle to a TensorFlow operation. That op
will initialize all the global variables when we run it with `tf.Session.run`.
Also note that this `global_variables_initializer` only initializes variables
that existed in the graph when the initializer was created. So the initializer
should be one of the last things added during graph construction.
### Executing Layers
Now that the layer is initialized, we can evaluate the `linear_model`'s output
tensor as we would any other tensor. For example, the following code:
```python
print(sess.run(y, {x: [[1, 2, 3],[4, 5, 6]]}))
```
will generate a two-element output vector such as the following:
```
[[-3.41378999]
[-9.14999008]]
```
### Layer Function shortcuts
For each layer class (like `tf.layers.Dense`) TensorFlow also supplies a
shortcut function (like `tf.layers.dense`). The only difference is that the
shortcut function versions create and run the layer in a single call. For
example, the following code is equivalent to the earlier version:
```python
x = tf.placeholder(tf.float32, shape=[None, 3])
y = tf.layers.dense(x, units=1)
init = tf.global_variables_initializer()
sess.run(init)
print(sess.run(y, {x: [[1, 2, 3], [4, 5, 6]]}))
```
While convenient, this approach allows no access to the `tf.layers.Layer`
object. This makes introspection and debugging more difficult,
and layer reuse impossible.
## Feature columns
The easiest way to experiment with feature columns is using the
`tf.feature_column.input_layer` function. This function only accepts
@{$feature_columns$dense columns} as inputs, so to view the result
of a categorical column you must wrap it in an
`tf.feature_column.indicator_column`. For example:
``` python
features = {
'sales' : [[5], [10], [8], [9]],
'department': ['sports', 'sports', 'gardening', 'gardening']}
department_column = tf.feature_column.categorical_column_with_vocabulary_list(
'department', ['sports', 'gardening'])
department_column = tf.feature_column.indicator_column(department_column)
columns = [
tf.feature_column.numeric_column('sales'),
department_column
]
inputs = tf.feature_column.input_layer(features, columns)
```
Running the `inputs` tensor will parse the `features` into a batch of vectors.
Feature columns can have internal state, like layers, so they often need to be
initialized. Categorical columns use `tf.contrib.lookup`
internally and these require a separate initialization op,
`tf.tables_initializer`.
``` python
var_init = tf.global_variables_initializer()
table_init = tf.tables_initializer()
sess = tf.Session()
sess.run((var_init, table_init))
```
Once the internal state has been initialized you can run `inputs` like any
other `tf.Tensor`:
```python
print(sess.run(inputs))
```
This shows how the feature columns have packed the input vectors, with the
one-hot "department" as the first two indices and "sales" as the third.
```None
[[ 1. 0. 5.]
[ 1. 0. 10.]
[ 0. 1. 8.]
[ 0. 1. 9.]]
```
## Training
Now that you're familiar with the basics of core TensorFlow, let's train a
small regression model manually.
### Define the data
First let's define some inputs, `x`, and the expected output for each input,
`y_true`:
```python
x = tf.constant([[1], [2], [3], [4]], dtype=tf.float32)
y_true = tf.constant([[0], [-1], [-2], [-3]], dtype=tf.float32)
```
### Define the model
Next, build a simple linear model, with 1 output:
``` python
linear_model = tf.layers.Dense(units=1)
y_pred = linear_model(x)
```
You can evaluate the predictions as follows:
``` python
sess = tf.Session()
init = tf.global_variables_initializer()
sess.run(init)
print(sess.run(y_pred))
```
The model hasn't yet been trained, so the four "predicted" values aren't very
good. Here's what we got; your own output will almost certainly differ:
``` None
[[ 0.02631879]
[ 0.05263758]
[ 0.07895637]
[ 0.10527515]]
```
### Loss
To optimize a model, you first need to define the loss. We'll use the mean
square error, a standard loss for regression problems.
While you could do this manually with lower level math operations,
the `tf.losses` module provides a set of common loss functions. You can use it
to calculate the mean square error as follows:
``` python
loss = tf.losses.mean_squared_error(labels=y_true, predictions=y_pred)
print(sess.run(loss))
```
This will produce a loss value, something like:
``` None
2.23962
```
### Training
TensorFlow provides
[**optimizers**](https://developers.google.com/machine-learning/glossary/#optimizer)
implementing standard optimization algorithms. These are implemented as
sub-classes of `tf.train.Optimizer`. They incrementally change each
variable in order to minimize the loss. The simplest optimization algorithm is
[**gradient descent**](https://developers.google.com/machine-learning/glossary/#gradient_descent),
implemented by `tf.train.GradientDescentOptimizer`. It modifies each
variable according to the magnitude of the derivative of loss with respect to
that variable. For example:
```python
optimizer = tf.train.GradientDescentOptimizer(0.01)
train = optimizer.minimize(loss)
```
This code builds all the graph components necessary for the optimization, and
returns a training operation. When run, the training op will update variables
in the graph. You might run it as follows:
```python
for i in range(100):
_, loss_value = sess.run((train, loss))
print(loss_value)
```
Since `train` is an op, not a tensor, it doesn't return a value when run.
To see the progression of the loss during training, we run the loss tensor at
the same time, producing output like the following:
``` None
1.35659
1.00412
0.759167
0.588829
0.470264
0.387626
0.329918
0.289511
0.261112
0.241046
...
```
### Complete program
```python
x = tf.constant([[1], [2], [3], [4]], dtype=tf.float32)
y_true = tf.constant([[0], [-1], [-2], [-3]], dtype=tf.float32)
linear_model = tf.layers.Dense(units=1)
y_pred = linear_model(x)
loss = tf.losses.mean_squared_error(labels=y_true, predictions=y_pred)
optimizer = tf.train.GradientDescentOptimizer(0.01)
train = optimizer.minimize(loss)
init = tf.global_variables_initializer()
sess = tf.Session()
sess.run(init)
for i in range(100):
_, loss_value = sess.run((train, loss))
print(loss_value)
print(sess.run(y_pred))
```
## Next steps
To learn more about building models with TensorFlow consider the following:
* @{$custom_estimators$Custom Estimators}, to learn how to build
customized models with TensorFlow. Your knowledge of TensorFlow Core will
help you understand and debug your own models.
If you want to learn more about the inner workings of TensorFlow consider the
following documents, which go into more depth on many of the topics discussed
here:
* @{$graphs}
* @{$tensors}
* @{$variables}
|